Pass Microsoft Certified: Azure Data Engineer Associate Certification Exam in First Attempt Guaranteed!
Get 100% Latest Exam Questions, Accurate & Verified Answers to Pass the Actual Exam!
30 Days Free Updates, Instant Download!
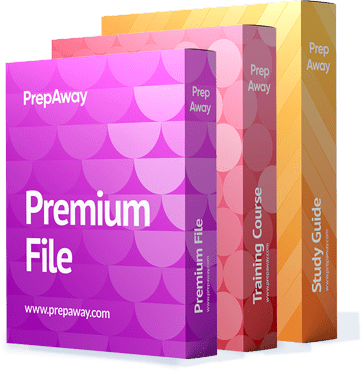
DP-203 Premium Bundle
- Premium File 397 Questions & Answers. Last update: Jun 09, 2025
- Training Course 262 Video Lectures
- Study Guide 1325 Pages
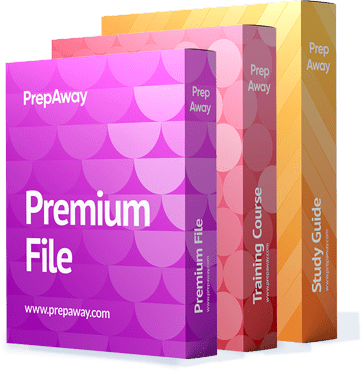
DP-203 Premium Bundle
- Premium File 397 Questions & Answers
Last update: Jun 09, 2025 - Training Course 262 Video Lectures
- Study Guide 1325 Pages
Purchase Individually
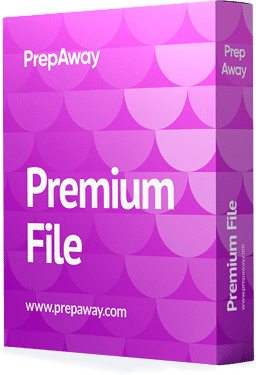
Premium File
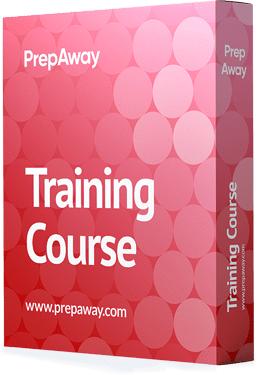
Training Course
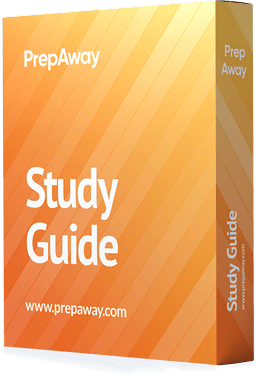
Study Guide
DP-203 Exam - Data Engineering on Microsoft Azure
Download Free DP-203 Exam Questions |
---|
Microsoft Microsoft Certified: Azure Data Engineer Associate Certification Practice Test Questions and Answers, Microsoft Microsoft Certified: Azure Data Engineer Associate Certification Exam Dumps
All Microsoft Microsoft Certified: Azure Data Engineer Associate certification exam dumps, study guide, training courses are prepared by industry experts. Microsoft Microsoft Certified: Azure Data Engineer Associate certification practice test questions and answers, exam dumps, study guide and training courses help candidates to study and pass hassle-free!
Microsoft Certified: Azure Data Engineer Associate certification practice test questions and answers, training course, study guide are uploaded in ETE files format by real users. Study and pass Microsoft Microsoft Certified: Azure Data Engineer Associate certification exam dumps & practice test questions and answers are the best available resource to help students pass at the first attempt.