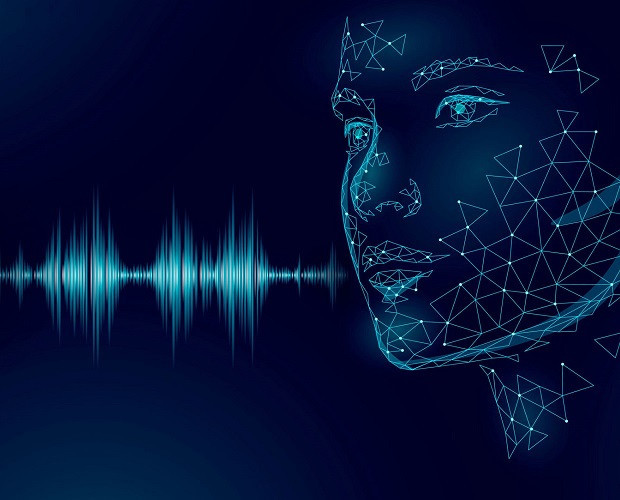
AWS Certified Machine Learning - Specialty: AWS Certified Machine Learning - Specialty (MLS-C01) Certification Video Training Course
The complete solution to prepare for for your exam with AWS Certified Machine Learning - Specialty: AWS Certified Machine Learning - Specialty (MLS-C01) certification video training course. The AWS Certified Machine Learning - Specialty: AWS Certified Machine Learning - Specialty (MLS-C01) certification video training course contains a complete set of videos that will provide you with thorough knowledge to understand the key concepts. Top notch prep including Amazon AWS Certified Machine Learning - Specialty exam dumps, study guide & practice test questions and answers.
AWS Certified Machine Learning - Specialty: AWS Certified Machine Learning - Specialty (MLS-C01) Certification Video Training Course Exam Curriculum
Introduction
-
1. Course Introduction: What to Expect
Data Engineering
-
1. Section Intro: Data Engineering
-
2. Amazon S3 - Overview
-
3. Amazon S3 - Storage Tiers & Lifecycle Rules
-
4. Amazon S3 Security
-
5. Kinesis Data Streams & Kinesis Data Firehose
-
6. Lab 1.1 - Kinesis Data Firehose
-
7. Kinesis Data Analytics
-
8. Lab 1.2 - Kinesis Data Analytics
-
9. Kinesis Video Streams
-
10. Kinesis ML Summary
-
11. Glue Data Catalog & Crawlers
-
12. Lab 1.3 - Glue Data Catalog
-
13. Glue ETL
-
14. Lab 1.4 - Glue ETL
-
15. Lab 1.5 - Athena
-
16. Lab 1 - Cleanup
-
17. AWS Data Stores in Machine Learning
-
18. AWS Data Pipelines
-
19. AWS Batch
-
20. AWS DMS - Database Migration Services
-
21. AWS Step Functions
-
22. Full Data Engineering Pipelines
Exploratory Data Analysis
-
1. Section Intro: Data Analysis
-
2. Python in Data Science and Machine Learning
-
3. Example: Preparing Data for Machine Learning in a Jupyter Notebook.
-
4. Types of Data
-
5. Data Distributions
-
6. Time Series: Trends and Seasonality
-
7. Introduction to Amazon Athena
-
8. Overview of Amazon Quicksight
-
9. Types of Visualizations, and When to Use Them.
-
10. Elastic MapReduce (EMR) and Hadoop Overview
-
11. Apache Spark on EMR
-
12. EMR Notebooks, Security, and Instance Types
-
13. Feature Engineering and the Curse of Dimensionality
-
14. Imputing Missing Data
-
15. Dealing with Unbalanced Data
-
16. Handling Outliers
-
17. Binning, Transforming, Encoding, Scaling, and Shuffling
-
18. Amazon SageMaker Ground Truth and Label Generation
-
19. Lab: Preparing Data for TF-IDF with Spark and EMR, Part 1
-
20. Lab: Preparing Data for TF-IDF with Spark and EMR, Part 2
-
21. Lab: Preparing Data for TF-IDF with Spark and EMR, Part 3
Modeling
-
1. Section Intro: Modeling
-
2. Introduction to Deep Learning
-
3. Convolutional Neural Networks
-
4. Recurrent Neural Networks
-
5. Deep Learning on EC2 and EMR
-
6. Tuning Neural Networks
-
7. Regularization Techniques for Neural Networks (Dropout, Early Stopping)
-
8. Grief with Gradients: The Vanishing Gradient problem
-
9. L1 and L2 Regularization
-
10. The Confusion Matrix
-
11. Precision, Recall, F1, AUC, and more
-
12. Ensemble Methods: Bagging and Boosting
-
13. Introducing Amazon SageMaker
-
14. Linear Learner in SageMaker
-
15. XGBoost in SageMaker
-
16. Seq2Seq in SageMaker
-
17. DeepAR in SageMaker
-
18. BlazingText in SageMaker
-
19. Object2Vec in SageMaker
-
20. Object Detection in SageMaker
-
21. Image Classification in SageMaker
-
22. Semantic Segmentation in SageMaker
-
23. Random Cut Forest in SageMaker
-
24. Neural Topic Model in SageMaker
-
25. Latent Dirichlet Allocation (LDA) in SageMaker
-
26. K-Nearest-Neighbors (KNN) in SageMaker
-
27. K-Means Clustering in SageMaker
-
28. Principal Component Analysis (PCA) in SageMaker
-
29. Factorization Machines in SageMaker
-
30. IP Insights in SageMaker
-
31. Reinforcement Learning in SageMaker
-
32. Automatic Model Tuning
-
33. Apache Spark with SageMaker
-
34. Amazon Comprehend
-
35. Amazon Translate
-
36. Amazon Transcribe
-
37. Amazon Polly
-
38. Amazon Rekognition
-
39. Amazon Forecast
-
40. Amazon Lex
-
41. The Best of the Rest: Other High-Level AWS Machine Learning Services
-
42. Putting them All Together
-
43. Lab: Tuning a Convolutional Neural Network on EC2, Part 1
-
44. Lab: Tuning a Convolutional Neural Network on EC2, Part 2
-
45. Lab: Tuning a Convolutional Neural Network on EC2, Part 3
ML Implementation and Operations
-
1. Section Intro: Machine Learning Implementation and Operations
-
2. SageMaker's Inner Details and Production Variants
-
3. SageMaker On the Edge: SageMaker Neo and IoT Greengrass
-
4. SageMaker Security: Encryption at Rest and In Transit
-
5. SageMaker Security: VPC's, IAM, Logging, and Monitoring
-
6. SageMaker Resource Management: Instance Types and Spot Training
-
7. SageMaker Resource Management: Elastic Inference, Automatic Scaling, AZ's
-
8. SageMaker Inference Pipelines
-
9. Lab: Tuning, Deploying, and Predicting with Tensorflow on SageMaker - Part 1
-
10. Lab: Tuning, Deploying, and Predicting with Tensorflow on SageMaker - Part 2
-
11. Lab: Tuning, Deploying, and Predicting with Tensorflow on SageMaker - Part 3
Wrapping Up
-
1. Section Intro: Wrapping Up
-
2. More Preparation Resources
-
3. Test-Taking Strategies, and What to Expect
-
4. You Made It!
-
5. Save 50% on your AWS Exam Cost!
-
6. Get an Extra 30 Minutes on your AWS Exam - Non Native English Speakers only
About AWS Certified Machine Learning - Specialty: AWS Certified Machine Learning - Specialty (MLS-C01) Certification Video Training Course
AWS Certified Machine Learning - Specialty: AWS Certified Machine Learning - Specialty (MLS-C01) certification video training course by prepaway along with practice test questions and answers, study guide and exam dumps provides the ultimate training package to help you pass.
Exploratory Data Analysis
12. EMR Notebooks, Security, and Instance Types
EMR builds upon that. However, with the EMR notebook, it's a similar concept to encephalin, but with more integration with AWS itself. So the notebooks are back up to S3, for one thing, and you can actually do things like provision entire clusters from within the notebook.
So you can spin up an EMR notebook, spin up an EMR cluster, and start a feeding task to that cluster, all from an EMR notebook. They're hosted within a VPC for security purposes, and you can only access this through an AWS console. You can use EMRnotebooks to create Apache Spark applications and run queries on them on your cluster. They support Python, Spark, SparkAR, and Scala, and it comes prepackaged with popular open-source graphics libraries from Anaconda to help you prototype your code, visualise your results, and perform exploratory data analysis with Spark data frames.
They're all hosted outside of the EMR cluster itself, and the notebook files are backed up to S Three.So that's how you can actually spin up a cluster from an EMR notebook. The notebook itself is not in your cluster. It turns out EMR notebooks also allow multiple users from your organisation to create their own notebooks, attach them to shared multitenant EMR clusters, and just start experimenting with Apache Spark.
Collaboratively, notebooks are provided at no additional charge to EMR customers. Let's talk about EMR security a bit as well. It uses IAM policies to grant or deny permissions and determine what actions a user can perform within Amazon EMR and other EWS resources. You can also combine IAM policies with tagging to control access on a cluster-by-cluster basis.
I am roles for EMR FS request to AmazonS Three allow you to control whether cluster users can access files from Amazon EMR based on user group or Emrfs data location. In Amazon S3, it also integrates with Kerberos, which provides strong authentication through secret key cryptography that ensures that passwords and other credentials aren't sent over the network in an unencrypted format. It also works with SSH Secure Socket Shell to give your users a secure way to connect to the command line on cluster instances.
SSH can also be used for tunnelling to view the various web interfaces that exist on your EMR cluster. For client authentication and IAM roles, you can use Kerberos or Amazon EC key pairs. Service Role Instance Profile and Service Linked Role Control in Amazon EMR How Amazon EMR is able to access other AWS services Each cluster in the Amazon EMR must have a service role and a role for the Amazon EC2 instance profile. IAM policies attached to these roles provide permissions for the cluster to interoperate with other AWS services on behalf of a user. There's also an auto scaling role you need if your cluster uses automatic scaling and service-linked roles are used at the service.
For Amazon, EMR has lost the ability to clean up Amazon EC2 resources. Some guidance on choosing instance types for your EMR cluster The masternode should probably be an M-4 large if there are less than 50 nodes on your entire cluster. If you have a larger cluster than that, you might want to go to an M-4 large for your core and task nodes; M-4 large is usually a good choice. However, if you have a lot of external dependencies, like a web crawler, maybe you could go down to a T-2 medium, and if you need more performance, then you could go to an extra large for compute-intensive applications.
Obviously, you'd want to go with a high CPU instance or use a high memory instance if you know ahead of time that you have a very database- or memory-caching-intensive application. You could also use cluster computer instances if you're doing things like NLP or machine learning; that also might be a good choice there. And finally, it seems like spot instances have come up a lot in these exams. Again, spot instances are a good choice. For task nodes, you would only use a spot instance on a core or a master node. If you're testing or very cost-sensitive, you're risking partial data loss by using a spot instance on a core or a master node. You generally want to stick to task nodes for spot instances.
13. Feature Engineering and the Curse of Dimensionality
Let's dive into the world of feature engineering. What exactly is feature engineering in the context of machine learning? Well, basically, it's the process of applying what you know about your data to sort of trim down the features that you're using, maybe create new features, or transform the features you have. What do I mean by features? Well, those are the attributes of your training data—the things that you're training your model with. Let's take an example. So let's say we're trying to predict how much money people make based on various attributes of the people. So your features in that case might be the person's age, their height, their weight, their address, what kind of car they drive, or any number of other things, right? Some of those things are going to be relevant to the things you're trying to predict, and some of them won't be. So the process of feature engineering is in part just selecting which features are important to what I'm trying to predict and choosing those features wisely. A lot of times, you need to transform those features in some way as well. Maybe the raw data isn't useful for the specific model you're using. Maybe things need to be normalised, scaled, or encoded in some specific way. Often, you'll have things like missing data in the real world. Often, you do not have complete data for every single data point. And the way that you choose to deal with that can very much influence the quality of the resulting model that you have. Also, sometimes you want to create new features from the existing ones that you have. Perhaps the numerical trends in the data that you have for a given feature are better represented by taking the log of it, the square of it, or something like that. Or maybe you're better off taking several features and combining them mathematically into one to reduce your dimensionality. This is all that feature engineering is about. You can't just take all the data you have and throw it into this big machine-learning hopper and expect good things to come out the other end. This is really the art of machine learning. This is where your expertise is applied to actually get good results out of it. It's not just a mechanical process where you follow these steps, take all the data you have, throw it into this algorithm, and see what predictions you make. That's what separates the good machine-learning practitioners from the bad ones. Those who can actually do feature engineering are, of course, the most successful and valuable in the job market.
And this isn't stuff that's generally taught, right? So this is largely a lot of stuff that is learned through experience and actually being out there in the real world and practising machine learning. And that's why this exam actually focuses a lot on it. The Machine Learning certification exam attempts to separate those who have experience in this field from those who do not. They're really trying to give people who actually know what they're doing and have real-world experience a very big edge on this exam. And well, I'm going to do my best to teach it to you here. It's not something that's generally covered, but there are some general best practises that, if you know them, will definitely help you out in this exam.
Why is feature engineering important in the first place? Well, it's about the curse of dimensionality. What do we mean by that? Well, like I said, you can't just throw every feature you have into the machine and expect good things to happen. Too many features can actually be very problematic for a few different reasons. For starters, there is at least sparse data. So again, come back to the example of trying to train a model on the attributes of people. There are hundreds of attributes about a person you could come up with, right?
As previously stated, what is your age, height, and weight, and what type of vehicle do you drive? How much money do you make? Where do you live? Who knows? Where did you go to college? The list goes on and on and on. And you can actually envision each person as an avatar in the dimensional space of all these features.
Okay, so stay with me here. Imagine, for example, that the only feature we have is a person's age. You could represent a person by plotting their age along a single axis, right? Going from zero to 1,000 or whatever Now we throw in another dimension, say their height. We have another dimension, another axis, that we have this vector pointing to that encodes both their age on one axis and their height on another, right? So now we have a two-dimensional vector.
Throw in a third dimension there—say, how much money they make. Now we have a vector in three dimensions, where one dimension is their age, one dimension is their height, and one dimension is how much money they make. And as we keep adding more and more dimensions, the available space that we have to work with just keeps exploding, right? This is what we call the "curse of dimensionality." So the more features you have, the larger the space that we can find a solution within.
And having a big space to try to find the right solution in just makes it a whole lot harder to find that optimal solution. So the more features you have, the more sparse your data becomes within that solution space, and the harder it is to actually find the best solution. So you're better off boiling those features down to the ones that matter the most. That will give you less sparse data and make it a lot easier to find the correct solution. Also, just from a performance standpoint, imagine trying to create a neural network that has inputs for every one of those features encoded in whatever way it needs. Right?
This neural network would have to be massive, extremely wide at the bottom, and probably extremely deep as well to actually find all the relationships between these many features. And it's just going to be ridiculously hard to get that convergence on anything.
So a big part of success in machine learning is not just choosing the algorithm or cleaning your data, but also choosing the data that you're using in the first place. That's what feature engineering is all about. Again, a lot of it comes down to domain knowledge and sort of using your common sense about what will work and what won't toward improving your model and just experimenting with different things and seeing what makes an effect and what doesn't, what helps and what hurts things. So a lot of it is just going back and forth with, "Does this feature help things?" No. Okay, we won't use it. Does this feature help things? No. Okay, try something else.
Now, you don't always have to guess. To be fair, there are some more principled ways of doing dimension reduction. So one of them is called PCA (principal component analysis). and we'll talk about that more in the modelling section of the course. But PCA is a way of taking all those higher dimensions, all those different features that you have, and distilling them down into a smaller number of features and a smaller number of dimensions. And it tries to do this in a way that preserves information as well as possible. So, I mean, if you have enough computational power to actually use PCA on a large set of features, that is a more principled way of distilling it down to the features that actually matter.
And the features you end up with aren't actually things you can put a label on. It's just artificially created features that capture the essence of the features that you started with. Another method is to use Kmeans clustering. What's nice is that these are both unsupervised techniques, so you don't have to actually train them on anything. You can just throw the feature data you have into these algorithms. It will boil down to a smaller set of dimensions that will work just as well, if not more closely. But again, more features are not better, at least not in terms of what we call the "curse of dimensionality." And that's one of the main reasons that we want to do feature engineering, and that's one of the main things you're going to be doing in that process.
14. Imputing Missing Data
So a big part of feature engineering is the imputation of missing data. What do you do when your data has missing data elements in it? This is what happens in the real world. For every observation you make, there's going to be some missing data points, more likely than not.
Well, a simple solution is just called "mean replacement." The idea is that if you have a missing attribute or feature within one of the rows of your data, just replace it with the mean from the entire column. And remember, we're talking about columns, not rows. Here. You want to take the mean of all the other observations of that same feature. It doesn't really make sense to take the mean of all the other features for that row, right? So "mean replacement" is all about taking the mean of that column and replacing all the empty values with that mean. So it's fast and easy.
Those are some of the positives of this approach. It also doesn't affect the mean or the sample size of your overall data set because you're just replacing missing dates with the mean; it won't affect the overall mean of the entire data set, which can be nice. Now, one nuance is that if you have a lot of outliers in your data set, which is also something you have to deal with when preparing your data, you might find that the median is actually a better choice than the mean. So if you have a data set of a bunch of people, and maybe one of those columns is income, and some people don't report their income because they think it's sensitive, you might have your mean skewed by a bunch of millionaires or billionaires in your data set.
So if you do mean imputation in that sort of situation where you have outliers, you might end up with an overly high or overly low value that you're using for replacement. So if you do have outliers that are skewing your mean, you might want to think about using the median instead. That will be less sensitive to those outliers. But generally speaking, it's not the best choice for imputation. First of all, it only works at the column level. So if there are correlations between other features in your data set, it's not going to pick up on those. So if there is a relationship between, say, age and income, that relationship is going to be totally missed. So you couldn't end up saying that a 10-year-old is making, you know, $50,000 a year because that's the mean of your data set. But it really doesn't make sense, right? I mean, a 15-year-old wouldn't be making that much money yet, so it's a very naive approach from that standpoint.
The other issue is that you can't really use it on categorical features. How do you take the mean of a categorical piece of data? That just doesn't make sense, right? Although you could use the most frequent value, using the most commonly seen category would be a reasonable thing to do. In that case, it's sort of in the same spirit as "mean replacement," but not really the same thing. Overall, though, it's not going to be a very accurate method. It's a very ham-handed attempt at doing imputations. So although it's quick and easy and has some advantages in practice, if someone is asking you, say, on a certification exam, what's the best way to do data imputation? Isn't it likely to mean replacement? It's also probably not just dropping the missing rows, although, as we've seen, sometimes that's a reasonable thing to do if you do have enough data such that dropping a few rows doesn't matter. If you don't have too many rows that contain missing data, that doesn't sound unreasonable.
The other thing, too, is that you want to make sure that dropping the rows that have missing data doesn't bias your data set in some way. What if there's an actual relationship between which rows are missing data and some other attribute of those observations? For example, let's say that we're looking at income. Again, there might be a situation where people with very high or very low incomes are more likely to not report it. So by removing or dropping all of those observations, you're actually removing a lot of people that have very high or low incomes from your model, and that might have a very bad effect on the accuracy of the model you end up with.
So you want to make sure that if you are going to drop data, it's not going to bias the data set in some way as a byproduct. Right? So it's a very quick and easy thing to do—probably the quickest and easiest thing to do. You can literally do this in one line of code in Python, but it's probably never going to be the best approach. So again, if an exam is asking you what's the best way to impute missing data, dropping data is probably not the right answer. Almost anything is going to be better. Maybe you could just substitute a similar field, right?
I mean, that would also be a simple way of doing it. For example, I might have a dataset of customer reviews of movies. Maybe if I have a review summary and a full text review as well, it would make more sense to just take the review summary and copy that into the full text for people who left the full text blank, for example. So almost anything is better than just dropping data. But in the real world, if you're just trying to do something quick and dirty, like start experimenting with some data just to play with it, it can be a reasonable thing to do. I just wouldn't leave that in place for production, necessarily. The thing you probably really want to do in production is use machine learning itself to impute your missing data into your machine learning training. So it's kind of a meta thing. There are different ways of doing this.
One is called KNN. This abbreviation stands for "K nearest neighbors." And if you have any experience with machine learning, you probably know what that is already. The general idea is to find K, where K is some number of the most similar rows to the ones that you're looking at that have missing data, and just average together the values from those most similar rows. So you can imagine having some sort of distance metric between each row. Maybe it's just the Euclidean distance between the normalised features within each row or something like that. And if you find the, say, ten nearest rows that are most similar to the one that's missing data, you can just take the average of that feature from those ten most similar rows and impute the value from that.
So that takes advantage of relationships between the other features of your data set, which is a good thing. One problem with it, though, is that this idea assumes that you have numerical data that you're trying to impute and not categorical data. It's difficult to take the average of a category, but there are techniques for doing so, such as hammering distance. But KN is generally a better fit for numerical data, not categorical data. If you have categorical data, you're probably better served by actually developing a deep learning model. Neural networks are great at solving categorization problems. So the idea is to actually build a machine learning model to impute the data for your machine learning model, right? It's kind of a cycle there, and that works really well for categorical data. It's really good.
It's tough to do deep learning these days. However, of course, it is complicated. There's a lot of code involved and a lot of tuning as well. But it's hard to predict the results if you actually have a deep learning model that tries to predict what a missing feature is based on other features in your data set. That's going to take a lot of work and a lot of computational effort, but it's going to give you the best results. You can also just do a multiple regression on the other features that are in your data set. That's also a totally reasonable thing to do. And through regressions, you can find linear or nonlinear relationships between your missing feature and the other features that are in your data set.
And there is a very advanced technique along these lines called "Mice," which stands for Multiple Imputation by Chained Equations. It's kind of the state of the art in this space right now for imputing missing data. So if you see a question that says, "What's a good way to impute missing data?" And one of the answers is "there's a good chance that might be a reasonable answer for you there"? To be clear, I didn't actually see a question like that in the exam, but if I were running the exam, I might put that on there. All right. And finally, probably the best way to deal with missing data is to just get more data. So if you have a bunch of rows that have missing data, maybe you just have to try harder to get more complete data from people.
And it's hard to just keep getting more real data so that you don't have to worry about all the rows that have missing data. Again, you want to be careful that if you're dropping data, you're not biassing your data set in some way. But really, the best way to deal with not having enough data is to just get more of it. Sometimes you just have to go back and figure out where that data came from and collect more, better-quality data. As a result, the higher the quality of data entering your system, the better the results. And while imputation techniques are a way to solve COVID issues where you just don't have enough data and can't get more of it, it's always a good idea to just get more and better data if you can.
15. Dealing with Unbalanced Data
Another problem in the world of feature engineering is handling unbalanced data. What do you mean by that? Well, that's when we have a large discrepancy between our positive and negative cases in our training data. So a common example is that in the world of fraud detection, actual fraud is pretty rare, right? So most of your training data is going to contain training rows that are not fraudulent. This can lead to difficulty in actually building a model that can identify fraud because there are so few data points to learn from compared to all of the non-fraud data points. So it's very easy for a model to say, "Okay, well, since fraud actually only happens like 0% of the time, I'm just going to predict that it's not fraud all the time, and hey, my accuracy is awesome now, right?" So if you have an unbalanced data set like that, you can end up in a situation like that where you have a machine learning model that looks like it has high accuracy, but it's just guessing no every time, and that's not helpful, right? So there are ways of dealing with this in feature engineering. Now, first of all, don't let the terminology confuse you. This is actually something that I got hung up on a lot at first. When I say positive and negative cases, I'm not talking about good and bad. As a result, don't confuse positive and negative with a positive and negative outcome. Positive simply means, "is this the thing that I'm testing for?" Is that what happened? So that might be fraud, right? So if my model is trying to detect fraud, then fraud is the positive case, even though fraud is a very negative thing.
Remember, positive is just the thing that you're trying to detect, whatever that is. So beat that into your head, because if you keep conflating positive and negative with, like, moral judgments, that's not what it's about in this context. By the way, this is mainly a problem with neural networks, by the way.
So it is a real issue that if you have an unbalanced data set like this, it's probably not going to learn the right thing, and we have to deal with that somehow. What's one way to deal with it? Just oversampling is a simple solution. So just take samples from your minority class in this example of fraud and take more of those samples that are known to be fraud and copy them over and over and over again. Make an army of clones, if you will, of your fraudulent test cases. and you can do that at random. You would think that that wouldn't actually help, but it does with a neural network.
So that's a very simple thing you can do. Just fabricate more of your minority case by making copies of other samples from that minority case. The other option is to use under sampling. So instead of creating more of your minority cases, remove the majority ones. So in the case of fraud, we'd be talking about just removing some of those non-fraudulent cases to balance it out a little bit more. However, throwing data away is usually not the right answer. Why would you ever want to do that?
You're discarding information, right? So the only time when under sampling might make sense is if you're specifically trying to avoid some scaling issue with your training. Maybe you just have more data than you can handle on the hardware that you're given. And if you have too much data to actually process and handle, by all means throw away some of the majority case. That might be a reasonable thing to do. But the better solution would be to get more computational power and actually scale this out on a cluster or something. So under sampling is usually not the best approach. Something that's even better than under sampling or oversampling is something called smoke. and this is something you might see. It stands for a synthetic minority oversampling technique—a kind of creative acronym. What it does is artificially generate examples of the minority class using its nearest neighbors.
So just like we talked about using KNN for imputation, the same idea applies here: we're running knee neighbors on each sample of the minority class, and then we create new samples from those KNN results by taking the mean of those neighbors. So instead of just naively making copies of other test cases for the minority class, we're actually fabricating new ones based on averages from other samples and fabricating them that way, which works pretty well. So it both generates new samples and under samples the majority class, which is good. So this is better than just oversampling by making copies because it's actually fabricating new data points that still have some basis in reality still. So remember, if you're dealing with unbalanced data, SMOTE is a very good choice.
A simpler approach, too, is just adjusting the thresholds when you're actually making inferences and applying your model to the data that you have. So when you're making predictions for a classification, say fraud or not fraud, you're going to have some sort of threshold of probability at which you say, "Okay, this is probably fraud." You know, most machine learning models don't just output "fraud" or "not fraud." It will actually give you a probability of whether or not it is a scam. And you have to choose a threshold of probability at which you say, "Okay, this is probably fraud." It deserves an investigation. So if you have too many false positives, one way to fix that is to just increase that threshold, right? So that is guaranteed to reduce your false-positive rate, but it comes at the cost of more false-negatives. So before you do something like this, you have to think about the impact that setting that threshold will have.
So if I raise my threshold, that means I'm going to have fewer actual things that are flagged as fraud. That might mean that I miss out on some actual fraudulent transactions there, but I'm not going to bother my customers as much by saying, "Hey, I flagged this as fraud; I shut down your credit card." You might actually want the opposite effect, right? Maybe I'll lower the threshold to get more fraud if I want to be more liberal about what I flag as fraud. Cases that are flagged as fraud might be ones where you're better off guessing wrong if it's not fraud than the other way around. Right. So you need to think about the cost of a false positive versus a false negative and choose your thresholds accordingly.
16. Handling Outliers
Yet another problem in the world of feature engineering is dealing with outliers in your data set. How do you handle them? Do you need to handle them? How do you identify them? So before we talk about outliers, we need a little bit of a mathematical background here. Don't worry, it's not hard. So let's start with the concept of variance.
How do you measure variance? We usually refer to it as "sigma squared," and you'll see why in a moment. But for now, just know that variance is the average of the squared differences from the mean. So to compute the variance of a data set, you first figure out the mean of it. So let's say I have some data that could represent anything. Let's say the maximum number of people that were standing in line for a given hour or something. And I saw one person standing in line for the first hour, then four, then five, then four, then eight. Okay? So the first step in computing the variance is to find the mean, the average of that data.
I just add them all together divided by the number of data points, and that comes out to 4.4 in this case as the average number of people standing in line. Now the next step is to find the differences from the mean for each data point. So I know the mean is four four. So I have one data point for my first,.44. So one minus four, four is negative three, four minus four, four is negative zero four, and so on and so forth. So I end up with these positive and negative numbers that represent the variance from the mean for each data point. Okay? But what I want is a single number that represents the variance of this entire data set.
So the next thing I'll do is find the squared differences. So we just go through each one of these raw differences from the mean and square them. This is for a couple of different reasons. First of all, I want to make sure that negative variances count just as much as positive variances. Otherwise, they'd all just cancel each other out, and that would be bad. I also want to give more weight to the outlier. So this amplifies the effect of things that are very different from the mean, all while still making sure that negative and positive variances are compared comparably. because the square of a negative is a positive, right? You always end up with a positive value when you square something.
So let's look at what happens when I do this. So negative three four squared, that's positive eleven, six; negative zero four squared is a much smaller number, 16, because that's much closer to the mean of four. With only 00:36 there, four and a half minutes is also closer to the mean. But as we get up to the positive outlier, 36, that ends up being 1296. And to find the actual variance value, we just take the average of all those squared differences from the mean, add up all those squared variances, divide by five, the number of values that we have, and we end up with a total variance of five and four. That's all variance. Let's move on to the standard deviation. Typically, we talk more about standard deviation than variance. And it turns out that the standard deviation is just the square root of the variance.
So I had a variance of five to four. The standard deviation is 224. So you can see why a variance is called sigma squared, because sigma represents the standard deviation. So if I take the square root of sigma squared, I get sigma, and it ends up in this example being 224. Now, this is a histogram of the actual data we were looking at. We see that the number four occurred twice in our dataset, and then we had one, five, and eight. Now, the standard deviation is usually used as a way to think about how to identify outliers in your data set. So if I'm within one standard deviation of the mean of four, that's considered to be a kind of typical value in a normal distribution.
But you can see in this example that the numbers one and eight actually lie outside of that range. So if I take four plus or minus 224, we end up around there and there, and one and eight both fall outside of that range of standard deviation. So we can say mathematically that one in eight are outliers. We don't have to guess and eyeball it. That has a mathematical foundation. There is still some discretion in determining what constitutes an outlier in terms of how many standard deviations it deviates from the mean. So, in general, the number of standard deviations from the mean indicates how much of an outlier or data point there is. So that's something you'll see standard deviation used for in the real world. And you might define your outliers as being one standard deviation away from the mean or two standard deviations away from the mean.
That's the kind of judgement call that you need to make as you're doing your feature engineering. So sometimes it's appropriate to remove those outliers once you've identified them, and sometimes it isn't. Make sure you make that decision responsibly. So, for example, if I'm doing collaborative filtering and I'm trying to make movie recommendations or something like that, you might have a few power users that have watched every movie ever made and rated every movie ever made. They could end up having an inordinate influence on the recommendations for everyone else. And you usually don't want a handful of people to have that much power in your machine learning model. So that might be an example where it would be a legitimate thing to filter out an outlier and identify them by how many ratings they've actually put into the system.
Or maybe an outlier would be someone who doesn't have enough ratings. Or you might be looking at web log data, where an outlier could be telling you that there's something very wrong with your data to begin with. It could be malicious traffic, or it could be bots or other agents that should be discarded because they don't represent the actual human beings that you're trying to model. But if someone really wanted the mean average income in the United States and not the median, they would specifically want the mean. And you shouldn't just throw out the billionaires in your country because you don't like them.
The truth is that there will be billions of dollars that will push that mean amount up even if it does not move the median. So don't fudge your numbers by throwing out outliers, but throw out outliers if they're not consistent with what you're trying to model in the first place. So how do we identify outliers? Well, remember our old friend, the standard deviation? We covered that a few slides ago. It's a very useful tool for detecting outliers. And it's extremely difficult to find the standard deviation of a data set. And if you see a data point that's outside one or two standard deviations, then you have an outlier. Remember the box and whisker diagrams that we talked about earlier too? Now those have built-in ways of detecting and visualising outliers, and those define outliers as lying outside the interquartile range. So what multiples do you choose?
Well, you kind of have to use common sense here. There's no hard-and-fast rule as to what constitutes an outlier. You have to look at your data, kind of eyeball it, look at the distribution, look at the histogram, see if there are actual things that stick out to you as obvious outliers, and understand what they are before you just throw them away. Also, in the context of AWS, AWS has an outlier algorithm of its own called random cut forest, and it's creeping up into more and more of their services. You can find random cut forests within QuickSite, Kinesis Analytics, Sage Maker, and more. So if you're talking about outliers and outlier detection, Amazon seems really, really proud of their random cut forest algorithms.
So there's a good chance that's what they're looking for in the exam. If they mentioned random cut forest when discussing outlier detection, Let's look at an example of income inequality. So let's say that we're modelling income distributions. We're just looking at how much money each person makes each year, and we're trying to understand what that means about the data set as a whole.
So in this simple example here, again, you're not going to be given Python code in the exam; this is just to make it real for you guys. We've set up a normal distribution here of income centred around $27,000, and we've added in a single billionaire and plotted the histogram of that. And you can see that that one billionaire really screwed up our distribution. So all of those ordinary people, all of the non-billionaires, have been crammed into a single line off to the left.
And our billionaire, that one little data point you can't even see, has skewed our data massively. And if we were to take the mean of this, we'd get a really wacky number. So a very simple outlier detection function has been written. All it does is compute the median of the entire data set, compute a standard deviation, and if anything lies outside of two standard deviations, it throws it out.
And if we call this function "reject outliers" and then plot the histogram, we get much more meaningful data by rejecting that outlier. We also have a more meaningful meaning now. That's closer to the $27,000 that we started with. So that's an example of where you want to think about where your outliers are coming from. Is it really appropriate to throw away that billionaire or not? What effect is that really having on the business outcome that you're trying to achieve at the end of the day?
Prepaway's AWS Certified Machine Learning - Specialty: AWS Certified Machine Learning - Specialty (MLS-C01) video training course for passing certification exams is the only solution which you need.
Pass Amazon AWS Certified Machine Learning - Specialty Exam in First Attempt Guaranteed!
Get 100% Latest Exam Questions, Accurate & Verified Answers As Seen in the Actual Exam!
30 Days Free Updates, Instant Download!
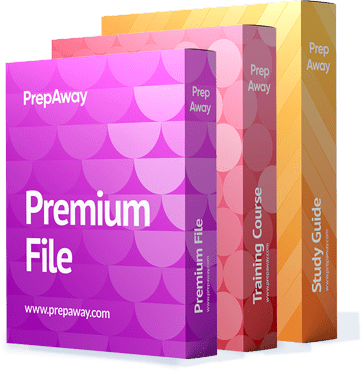
AWS Certified Machine Learning - Specialty Premium Bundle
- Premium File 332 Questions & Answers. Last update: Jul 17, 2024
- Training Course 106 Video Lectures
- Study Guide 275 Pages
Student Feedback
Can View Online Video Courses
Please fill out your email address below in order to view Online Courses.
Registration is Free and Easy, You Simply need to provide an email address.
- Trusted By 1.2M IT Certification Candidates Every Month
- Hundreds Hours of Videos
- Instant download After Registration
A confirmation link will be sent to this email address to verify your login.
Please Log In to view Online Course
Registration is free and easy - just provide your E-mail address.
Click Here to Register
IT Certification Tutorials
- Top Career Opportunities for Financial Certified Professionals
- Top Project Management Certifications to Improve Your CV
- Top 10 Computer Job Titles That Will Rule the Future
- Discontinuation of ITIL v3 in 2022 And New Technological Era
- GAQM CSM-001 Certified Scrum Master - Chapter 04 - Meetings in Scrum Part 3
- Python Institute PCAP - Modules; Packages and Object Oriented Programming in Python Part 3
- PMI PMP Project Management Professional - Introducing Project Risk Management Part 3
- CompTIA CASP+ CAS-004 - Chapter 01 - Understanding Risk Management Part 3
- DA-100 Microsoft Power BI - Part 2 Level 2 - Getting Multiple files
- CompTIA CASP+ CAS-004 - Chapter 04 - Implementing Security for Systems; Applications; and Storage Part 3
- IIBA CBAP - Tasks of Business Analysis Planning and Monitoring
- MB-210 Microsoft Dynamics 365 - Create and Manage Product and Product Catalog Part 2
- Salesforce Certified Platform App Builder - 5 - Business Logic and Process Automation Part 3
- Amazon AWS Certified Data Analytics Specialty - Domain 4: Analysis
- Google Professional Cloud Network Engineer - Designing; Planning; and Prototyping a GCP Network Part 3